Portfolio project
Dataiku global content
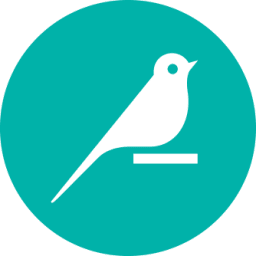
Moving from democratized data analytics to ‘everyday’ AI
Florian Douetteau, co-founder and CEO, Dataiku
The data democratization movement was underway long before the pandemic. Large enterprises were realizing the value in putting AI in the hands of many, not the elite few. That is, enabling all people – regardless of their background, profile, or role – to gain insights from data to work faster and smarter, together.
As we enter a new year we’re going to see data democratization evolve as the rise of the data economy gathers pace. In a post-pandemic world, the ability to be responsive, agile, and nimble will be the baseline for business and this means moving from democratized data analytics to ‘everyday AI’.
Devolution of data analytics
This delegation of power is because the notion of organizational-wide access, understanding and ability-to-act on data is now business-critical. According to Experian’s 2021 Data experience research report, 92% of business leaders have invested in data management in the last 12 months while 85% say they are increasingly focused on the data experience to become more data-driven as a business. Gartner found, in its 2021 Chief Data Officer Survey, that those who successfully increased data sharing led data and analytics teams that were 1.7 times more effective at showing demonstrable, verifiable value to stakeholders. The analysts group also predicts that by 2023, organizations that promote data sharing will outperform their peers on most business value metrics.
As a result, we will see automation, business intelligence, and AI converge into one practice, rather than simply being interconnected entities. This will fuel the proliferation of enterprise-wide “citizen data scientists”. With self-service data initiatives and platforms that enable AI democratization, it will no longer be the default to rely on data scientists or engineers for analysis. Today, there’s no need to wait for massive amounts of data to be pre-processed and go through complex data cleaning pipelines in order for it to be leveraged and used to inform decisions and unearth new, value-added opportunities for the organization. This will be devolved to a much more widespread level making analysis and decision-making the scope of more individuals in the organisation, more frequently – everyday, in fact.
When Everyday AI becomes a game-changer
But to realize this vision data democratization must evolve in the same way the supporting technology and field of skills and experience has. Without the right processes in place, data democratization can still lead to the creation of silos, and suddenly making data tools available across the business doesn’t mean everyone quickly becoming data experts. There is still considerable room for error.
To be transformative, organizations need agility and that comes from making the right decisions fast. But the amount of data can be overwhelming and difficult for many organisations to handle. At best organizations have a small number of highly technical data experts supporting large teams across the business. Often these data experts are busy working on issues with the highest priority, yet people all across the business still need help to solve contextual problems that require some data science but don’t justify – or can’t wait for – a data scientist. That means critical business decisions are being made daily that aren’t supported by the centralized data science team. This is where the concept of everyday AI becomes a game changer.
Better results when combining working practices
Foundational to this is the operational processes that underpin data and AI. Embedding the right tools, training, and knowledge-sharing principles will foster a considerable change in working practices. As a result, colleagues will look at how they engage with data and AI in new ways. With more opportunities for open collaboration – based on making data everyday – the right tools reduce the risk of siloes developing. With growing confidence, AI tools will become an even greater part of a business’ strategic agenda rather than an additional nice-to-have.
In something of a self-fulfilling prophecy, by doing the above, organizations will automatically broaden the sources of data that are being used. While small data often means siloed departments working with the data they collect, using larger datasets and more advanced tools allows analysts to break down the barriers between departments (within the limits of established governance and data privacy guidelines, of course) and even bring in third-party data (i.e., data from sources outside the company — think weather, social media data, etc.). The equation is a simple one – more data and more people involved combined with better tools and training equals better results.
Cultivating and curating citizen data scientists
As data analytics becomes more democratized, we’ll start seeing a bigger, more diverse roster of people with ‘normal’ job titles that have data and analytics as part of their day-to-day. Companies will start cultivating and curating these citizen data scientists to help them cut costs and reduce risk as AI is normalized and integrated into more and more job functions, roles, and responsibilities.
Data scientists are a critical piece of organizational data and AI transformation. They are – and will remain – the experts in this area and are leading the charge when it comes to upskilling but will only be able to handle so much given increased adoption and the sheer scale of enterprise AI initiatives. So while citizen data scientists certainly won’t replace traditional data scientists, the role of IT operators, domain experts, and even risk managers will be enriched as they take on the AI mantle to provide additional value helping organizations move from democratized data analytics to ‘everyday’ AI.